
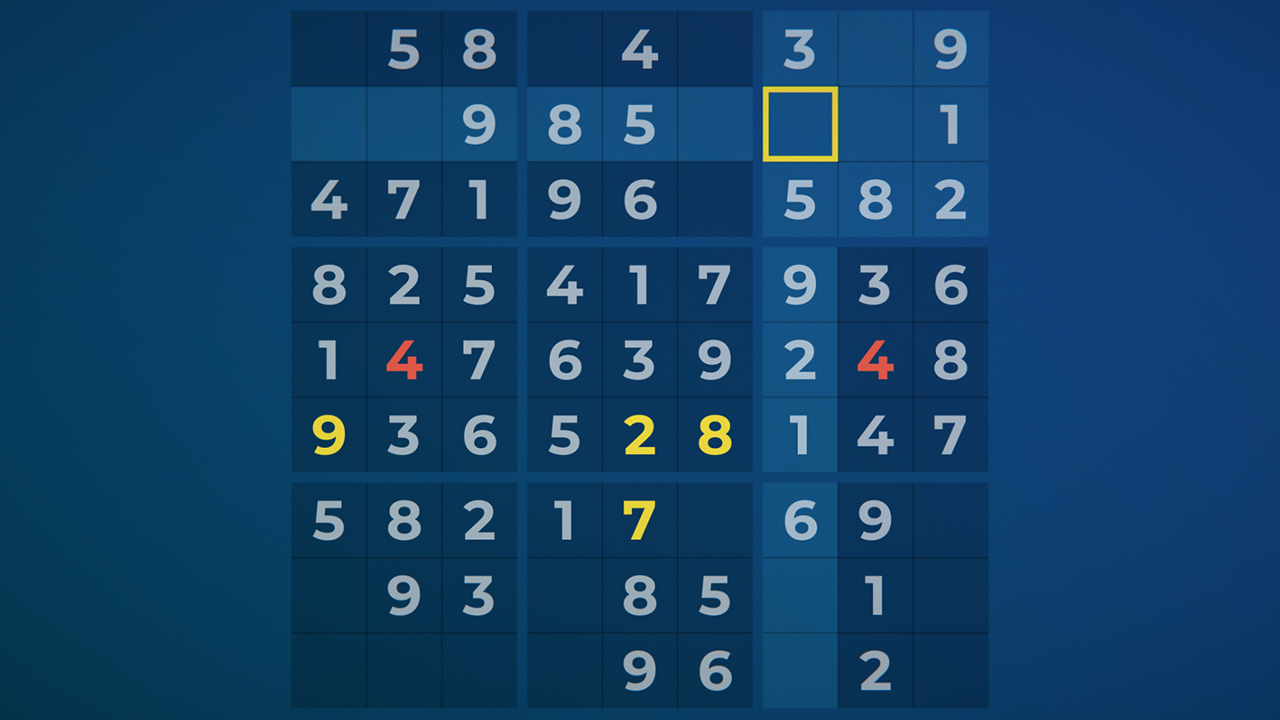
Our entirely automatic approach uses machine learning, specifically Global Vectors for Word Embeddings (GloVe), on a corpus collected from a social media healthcare platform to extend and enhance consumer health vocabularies. In this paper, we present an automatic method to enrich laymen’s vocabularies that has the benefit of being able to be applied to vocabularies in any domain.
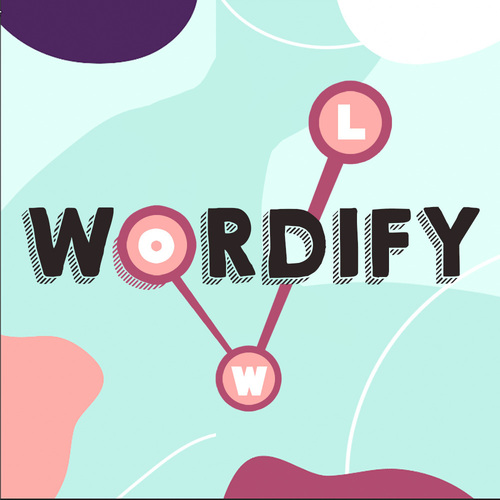
Many of the presented vocabularies are built manually or semi-automatically requiring large investments of time and human effort and consequently the slow growth of these vocabularies.
#Wordify numbers professional#
To bridge this gap, several professional vocabularies and ontologies have been created to map laymen medical terms to professional medical terms and vice versa. In healthcare, it is rare to find a layman knowledgeable in medical terminology which can lead to poor understanding of their condition and/or treatment. A layman may have difficulty communicating with a professional due to not understanding the specialized terms common to the domain. A discussion is also provided on the use of Wordify in conjunction with other text-analysis tools, such as probabilistic topic modeling and sentiment analysis, to gain more profound knowledge of the role of language in consumer behavior.Ĭlear language makes communication easier between any two parties. We show empirically that Wordify’s RLR algorithm performs better at discriminating vocabularies than support vector machines and chi-square selectors, while offering significant advantages in computing time. We present illustrative examples to show how the tool can be used for such diverse purposes as 1) uncovering the distinctive vocabularies that consumers use when writing reviews on smartphones versus PCs, 2) discovering how the words used in Tweets differ between presumed supporters and opponents of a controversial ad, and 3) expanding the dictionaries of dictionary-based sentiment-measurement tools. The tool, Wordify, uses randomized logistic regression (RLR) to identify the words that best discriminate texts drawn from different pre-classified corpora, such as posts written by men versus women, or texts containing mostly negative versus positive valence.
#Wordify numbers free#
If (finlOutPut != "dontAddBigSufix") )( NUMBER2TEXT.ones, NUMBER2TEXT.tens, NUMBER2TEXT.This work describes and illustrates a free and easy-to-use online text-analysis tool for understanding how consumer word use varies across contexts. function return the string "dontAddBigSufix" conver each grup of three digits to english word
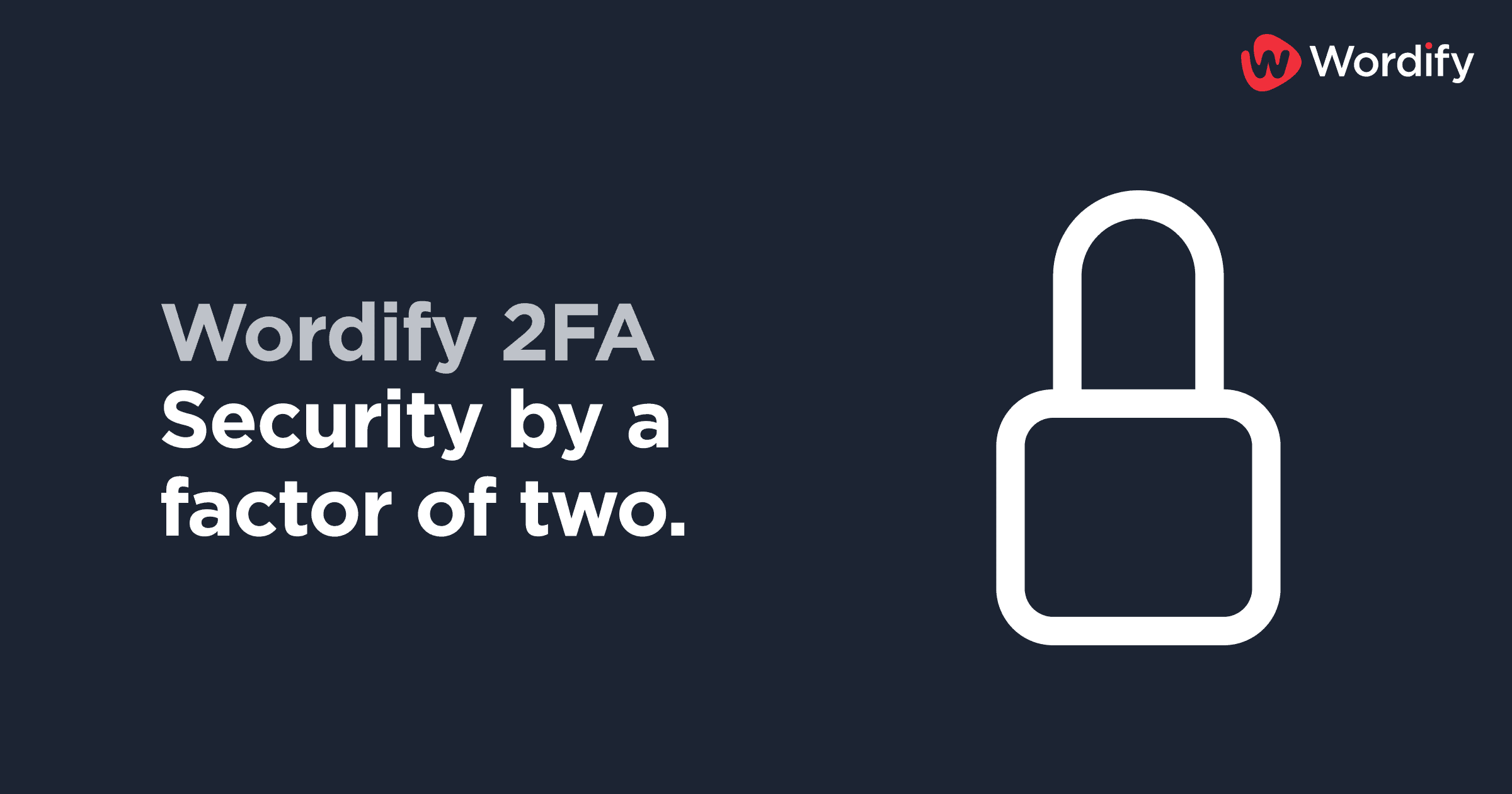
NumString = numString.substring(0, numString.length - 3) TriDig = numString.charAt(numString.length - 3) TriDig = numString.charAt(numString.length - 2) TriDig = numString.charAt(numString.length - 1) cut the number to grups of three digits and add them to the Arry Var numString = document.getElementById('number').value ĭocument.getElementById('container').innerHTML = 'Zero' ĭocument.getElementById('container').innerHTML = 'messeg tell to enter numbers' Var bigNumArry = new Array('', ' thousand', ' million', ' billion', ' trillion', ' quadrillion', ' quintillion') What did I do wrong? Where is the bug? Why does it not work perfectly? Somehow it "forgets" the last numbers when there are a few zeros. It seems to work pretty well, but I've got some problems with numbers like 19000000 9, converted to: " one hundred ninety million". If the finlOutPut cell equals "dontAddBigSufix" (because it was only zeroes), don't add the word and set the cell to " " (nothing). If all the three digits are zero convert them to "dontAddBigSuffix"įrom Right to left, add thousand, million, billion, etc. Separate the digits to three and put them on Array ( finlOutPut), from right to left.Ĭonvert each group (each cell in the finlOutPut array) of three digits to a word (this what the triConvert function does). I'm trying to convert numbers into english words, for example 1234 would become: " one thousand two hundred thirty four".
